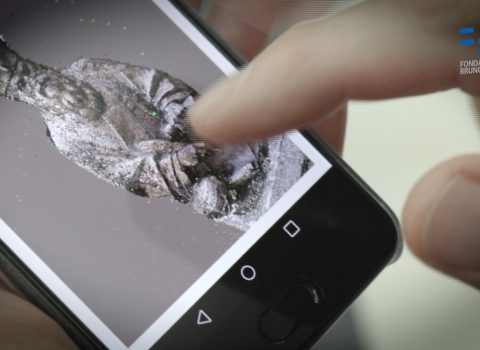
Big data, big faith? About beliefs and biases in algorithmic decision-making
With the arrival of Big Data analytics, data rapidly became the petroleum of the 21st century and a new social contract came into existence.
After all, as Alex Preston (2014) wrote: “Google knows what you’re looking for. Facebook knows what you like. Sharing is the norm, and secrecy is out”. Big Data and algorithms are everywhere, which is not a problem by itself. With many regards, these technologies are making our social and professional lives infinitely easier. Nevertheless, far from being objective, neutral and infallible, algorithms – those “constantly changing, theory laden, and naturally selective human artifacts produced within a business environment” (Balazka and Rodighiero, 2020) – can reproduce existing discriminations in a digital form.
This is not a new story. Despite a popular narrative claiming otherwise, there is nothing “raw” about data – whether big or small. There is the risk to misrepresent ethnic or religious minorities, to privilege men over women or to systematically harm frequently marginalized social actors like the LGBT community. With Big Data and algorithmic decision-making becoming more and more pervasive day by day, the social relevance of these issues grows.
Big Data and algorithms have real consequences. There is the well-known case of the flu tracker that consistently and repeatedly overestimated flu cases in the US (Lazer et al., 2014). More recently, 100 Colorado drivers trusted their online navigator a little bit too much ending up stuck in the mud (Lou, 2019). Far from being rare or isolated cases, these events are relatively common. To name just a few of the examples reported by the media in recent years, think about the “unconstitutional” algorithm that mishandled the transfers of over 10,000 teachers in Italy (Zunino, 2019), about racial and gender biases in job advertising (Biddle, 2019), or about recent arrests of Robert Julian-Borchak Williams (Hill, 2020) and Michael Oliver (O’Neill, 2020) caused by an error of the facial recognition algorithm employed by the police in Michigan. From biases in job recruitment (O’Neil, 2016) to manipulations of school’s grading procedure during the pandemic (Smith, 2020), algorithms sometime privilege specific sub-populations and/or those who know how to exploit the existing system to increase their visibility.
In the midst of Big Data hype, Chris Anderson (2008) announced the arrival of the “Petabyte Age” exploring the ways Big Data industry and its business model can enhance science. In the following years, we observed a redefinition of power dynamics in knowledge discovery due to the growing empowerment of non-academic, private and collective actors of various nature involved in the collection of Big Data. Over a decade later, it is arguably time to change the perspective and ask ourselves: what can the Big Data industry learn from science? In this sense, it is important to progressively shift the attention to the development of accountable, inclusive and transparent tools of decision-making (Jo and Gebru, 2020; Lepri et al., 2018) in order to counter digital stereotypization and account for biases based on gender, skin color, age, religion, sexual preferences and so on. To do so, we need to focus on the collection of better rather than just bigger data.
While instances of Big Data failures, or “data ops”, are everywhere around us, the belief in the accuracy and in the objectivity of Big Data analytics seems to be unshakable among many data outsiders (see Baldwin-Philippi, 2020). As Daniel and Richard McFarland (2015) pointed out, with Big Data there is a real “danger of being precisely inaccurate”, but such a danger is frequently overlooked outside of academic circles. From nowcasting to data informed decision- and policy-making, Big Data carries the promise of a deeper understanding of our social and physical surroundings and opens the door to the re-enchantment, not with magic or religion in its traditional form, but with a ‘datafied’ reality.
In a presumably secularized world where non-religion is progressively becoming a majoritarian phenomenon, Big Data is increasingly turning into a matter of faith. When precision, objectivity and accuracy become a belief rather than a subject of critical scrutiny, when results are accepted as implicitly and unquestionably true, then the World Wide Web becomes the ultimate prophet of a “pseudo omniscient algorithmic deity” (Gransche, 2016). Religion is not only about religion, but it can be a matter of nominal identity based on natal, ethnic or aspirational reasons (Day, 2013). Similarly, non-religion is not always about its absence, but can involve a variety of religious, spiritual, supernatural or secular beliefs (Balazka, 2020; Bullivant et al., 2019). And what about Big Data, machine learning and algorithms? Are they really always and only about hard science?
The author, Dominik Balazka, is a researcher at FBK-ICT and FBK-ISR. In collaboration with Smart Community Lab and Center for Religious Studies, he is currently working on a project about religious nones and (non)religious beliefs in the West. His research focuses on non-religion and on the impact of Big Data on the field of non-religion studies.