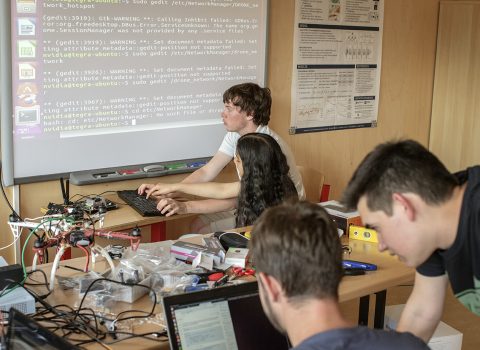
Do machines learn?
Marco Cristoforetti, a researcher at FBK's MPBA Unit who prepared a space at the Rimini Meeting, speaks of the important role played by machines and machine learning techniques in biomedical applications. With a look at ethics and the future
The Rimini Meeting, which took place August 20 through 26, saw the participation of two researchers from Fondazione Bruno Kessler: Cesare Furlanello, head of the MPBA (Predictive Models for Biomedicine and the environment) Research Unit and Marco Cristoforetti, a researcher of the team. In particular, Cristoforetti was among the organizers of the space “What? Machines That Learn”, setting up two meetings: the first, focusing on the topic of bio-pharmacological research and “machine learning”, featured Furlanello and Eugenio Aringhieri, CEO of the Dompé Group and Chairman of the Biotechnology Group of Farmindustria. The second, entitled “Do machines learn?”, was enlivened by Mauro Ceroni, a professor of neurology at the University of Pavia, and Pietro Leo, scientific director for Innovation, Technology and Research at IBM Italia.
Cristoforetti, a theoretical physicist, works as a data scientist in several projects concerning the application of machine learning to complex systems, particularly in biomedicine.
Marco, what were the most interesting ideas emerged during the meetings?
Particular attention has been paid to the application of machine learning in medicine, especially in the so-called precision medicine, based on individual differences, for example, using genetic variability or microbiome characteristics to diagnose and treat patients.
On the other hand, the volume of digital data is growing steadily, and this allows, on the one hand, to use machine learning techniques much more easily than before, while on the other it makes it almost essential, because working by hand becomes very complicated. For example, Pietro Leo pointed out that if a radiologist needs to see 4000 X-rays in one day, he or she is likely to get tired and lose efficiency: so it is obvious that if a computer does at least one first screening, energy is saved and the whole system becomes more effective. Also Aringhieri, from the point of view of drugs producers, emphasized the need to resort to these tools given the large amount of data available.
I must say that the response of the public was very positive. The audience was very heterogeneous, ranging from high school kids and older adults interested in the subject, but not working in the industry, to real experts. A very lively debate came out with so many questions from the public.
An interesting aspect is that of multidisciplinarity: research in this area involves simultaneously physicians, physicists, mathematicians and computer scientists. Is it a difficult coexistence?
There is definitely work to do. These are very different worlds that should seek more and more contamination, even at the level of training. A physician who has these tools available, and wants to use them effectively, should be able to handle them: nowadays everything is based on interaction, but the best solution for tomorrow would be for the physician to understand and analyze the data. At the same time, when developing our models, we should always keep in mind that the code we write is not everything: we always need to understand what the question we want to answer is precisely and then to interface with the physiian.
Another delicate issue is represented by the ethical and social implications of machine learning. Did you talk about this during meetings?
Yes, especially in the second meeting. One of the most recurring questions was “how far can a machine go?“, which touches the critical issue of liability: is there a limit to what I can delegate to the machine, partly giving up control? And also, “Will there ever be a machine capable of developing self-awareness?”
These are issues that very much appeal to the public, also because of the role played by the media, which often present these aspects in a sensationalistic way: people rightly begin to ask these questions both in a positive and a negative sense.
However, the issue is definitely premature: as Pietro Leo pointed out, today we are still far from such an advanced scenario. Machines are definitely capable of learning certain specific and well-defined tasks but are not yet able to make connections and put together different things. For example, a machine can now answer a specific question, but it is not yet able to ask itself questions, a task that requires far superior skills.
That said, it should also be emphasized that even if we were able to build machines capable of doing everything, we do not even know to what extent this would be really interesting and beneficial for us.
Where will machines arrive in the future?
I honestly do not know, this is an open problem. Certainly the way is still very long, and it makes one smile to think that in the 1950s, in the early stages of artificial intelligence, at some point it was thought that it was enough to close up ten scientists in a room for a month to get to conceive and build a machine that can behave like a human. In fact, only now, 60 years later, we are beginning to look with confidence in the development of artificial intelligence.