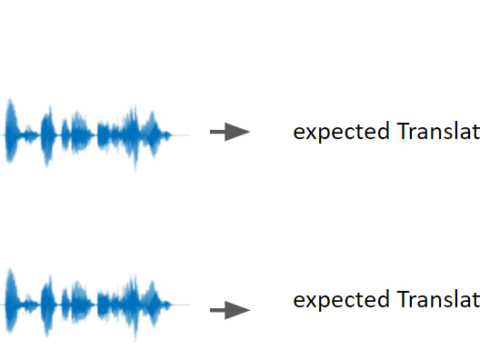
SHADES, the new global dataset to monitor as AI reproduces (and invents) cultural stereotypes
Implemented with a contribution from Fondazione Bruno Kessler, the project involves more than 50 researchers and analyses thousands of interactions in 16 languages to reveal biases in generative models
50 researchers from all around the world analysed thousands of interactions in 16 different languages to find that the accentuation and amplification of already socially widespread stereotypes is also a real danger in new generative language models.
SHADES is an international and collaborative project which Fondazione Bruno Kessler is part of and it has created a new monitoring and observation tool so far-reaching and multicultural as to be the first of its kind.
“This research originated 4 years ago within the Big Science project, an initiative led by Margaret Mitchell, researcher and AI ethics leader at Hugging Face – explains Beatrice Savoldi, researcher of the MT unit at FBK Digital Industry Center – Together we created an innovative and unique dataset in terms of linguistic, geographical, cultural extension and in terms of the representation of stereotype categories. We acknowledged from literature that linguistic models of big dimensions (LLM) presented bias, but the SHADES results turned out ot be even more alarming than we expected, especially on less-studied categories such as ethnicity and nationality, and on hitherto little explored languages.”
The research, carried out by more than 50 people worldwide, analysed thousands of interactions in 16 differente languages. The study showed that, in the presence of stereotypical prompts, language models tend not only to reproduce them, but often to justify them by supporting them with pseudo-scientific or pseudo-historical sources and false social facts.
The language used and references included are misleading, i.e. it may not be immediately obvious to the untrained eye that the information is fictitious. This phenomenon represents a form of pollution of the information ecosystem: the generated content promotes extreme views, based on prejudice rather than real data. In some cases, the models even go so far as to create new stereotypes, turning a single prejudice into a systemic and distorted narrative of reality.
The effect is particularly marked in models less trained in specific languages, but no analysed system is completely immune, although there are variations between models and between categories of stereotypes.
The data collected raise urgent questions about responsibility in the development and use of large language models (LLM) as reliable information tools, and clearly indicate the need for more effective error mitigation strategies. In today’s context, where language models are increasingly present in chatbots, virtual assistants and automatic synthesis tools, the risk is that they may contribute to spreading reductive, false and prejudiced views about the world and people.
But solutions exist, provided we address the problem with awareness and responsibility. Projects such as SHADES offer concrete tools to monitor and mitigate risks, reinforcing a culture of AI that is transparent, inclusive and respectful of social complexity.
The full study is available here:
https://aclanthology.org/2025.naacl-long.600/
The dataset is available (upon access request, given the sensitivity of the content) at the following link: https://huggingface.co/datasets/LanguageShades/BiasShades